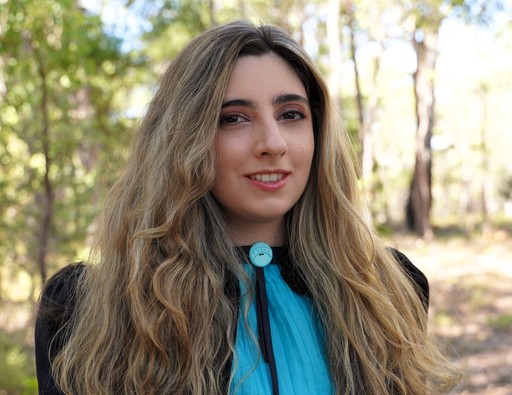
I develop artificial intelligence and machine learning (AI/ML), as well as mathematical and computational technologies, for biology and health. I am a Research Fellow in Biological Data Science at the University of Melbourne, and was previously a Doctoral Researcher at the Finnish Center for Artificial Intelligence (FCAI).
About Me
About Me
RESEARCH
AI in biotech & healthtech
My research develops AI/ML and mathematical and computational technologies for data-driven innovation in biology and health
My research develops AI/ML and mathematical and computational technologies for data-driven innovation in biology and health
Learn More
Learn More
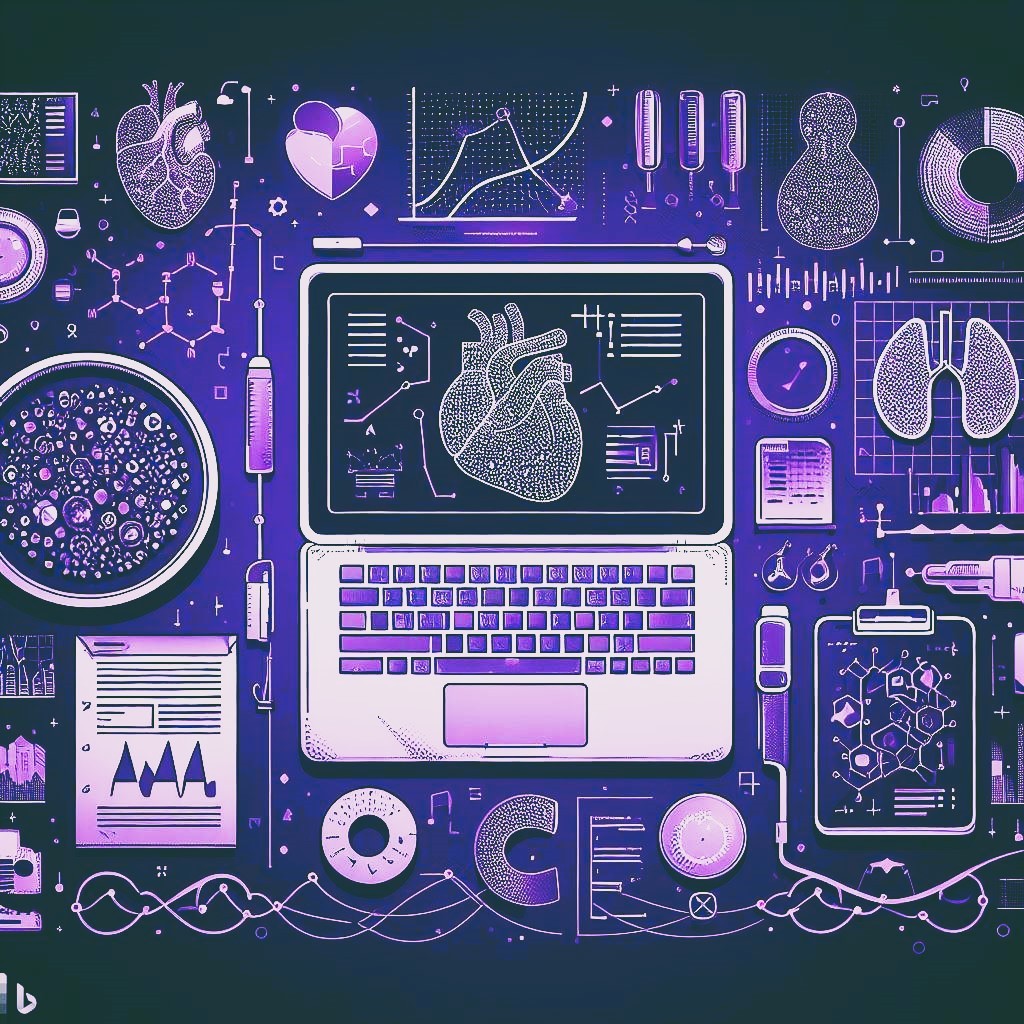
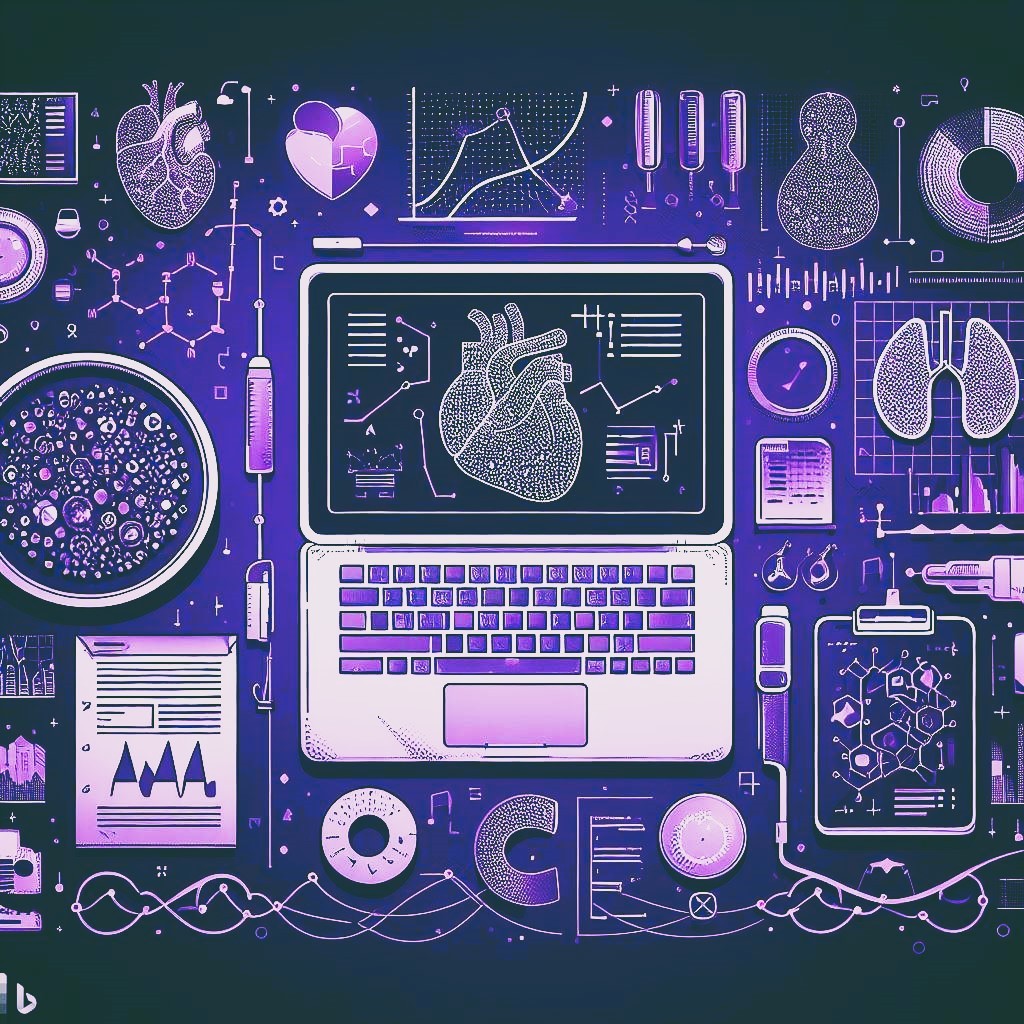
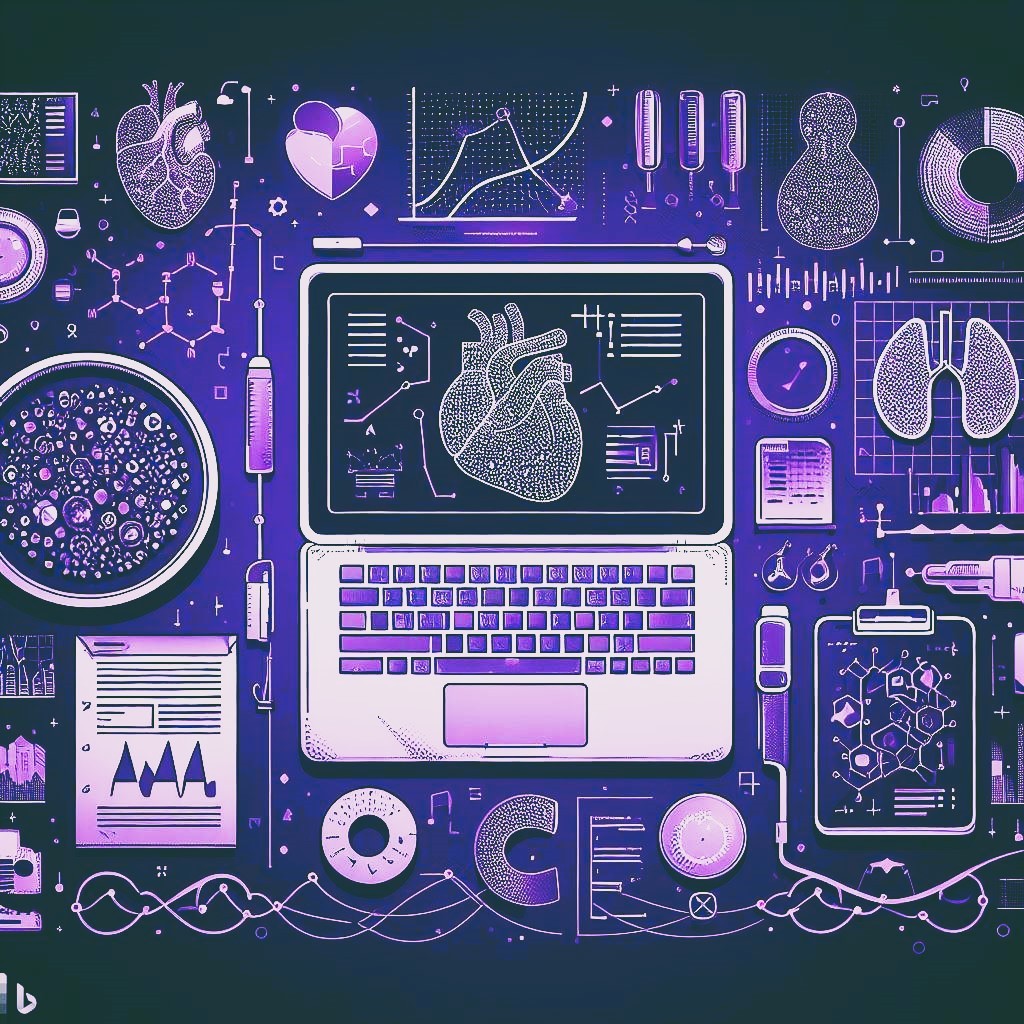
research highlights
Improving GeneraliSability of Health Prediction Models
Machine learning is a powerful tool for health prediction, but often struggles with generalisability challenges when making predictions for new patients. A promising strategy is to pool learning across related supervised learning tasks. A new Bayesian meta-learning approach is introduced that also models similarity between causal mechanisms of the tasks. This is applied to a case study for stroke prediction for health record and genetics data from the UK Biobank and FinnGen datasets.
Learn More
Published in
Preprint, Under review
Keywords
Meta-learning, Bayesian Hierarchical models, causal inference, deep learning, transfer learning, oOD generalisation, robust mL
Improving GeneraliSability of Health Prediction Models
Machine learning is a powerful tool for health prediction, but often struggles with generalisability challenges when making predictions for new patients. A promising strategy is to pool learning across related supervised learning tasks. A new Bayesian meta-learning approach is introduced that also models similarity between causal mechanisms of the tasks. This is applied to a case study for stroke prediction for health record and genetics data from the UK Biobank and FinnGen datasets.
Learn More
Published in
Preprint, Under review
Keywords
Meta-learning, Bayesian Hierarchical models, causal inference, deep learning, transfer learning, oOD generalisation, robust mL
Modeling disease risk in families with graph neural networks
Electronic health records (EHRs) spanning multiple generations present a new way for examining health trends in families. In collaboration with the Institute of Molecular Medicine Finland, an AI system was developed to analyze a network of over 7 million patients’ EHR data. The findings demonstrate that a geometric deep learning approach is beneficial for modeling the shared genetic, environmental, and lifestyle factors influencing disease risk in families.
Learn More
Published in
Machine Learning for Healthcare Conference (MLHC)
Keywords
graph neural networks, geometric deep learning, deep learning for sequential data, electronic health records, genetics, familial factors of disease
Modeling disease risk in families with graph neural networks
Electronic health records (EHRs) spanning multiple generations present a new way for examining health trends in families. In collaboration with the Institute of Molecular Medicine Finland, an AI system was developed to analyze a network of over 7 million patients’ EHR data. The findings demonstrate that a geometric deep learning approach is beneficial for modeling the shared genetic, environmental, and lifestyle factors influencing disease risk in families.
Learn More
Published in
Machine Learning for Healthcare Conference (MLHC)
Keywords
graph neural networks, geometric deep learning, deep learning for sequential data, electronic health records, genetics, familial factors of disease
Synthetic data FOR GENETICS RESEARCH
HAPNEST is a new software tool that efficiently generates large synthetic datasets that closely mimic real genetics and phenotypic data. This work was carried out with the European-wide INTERVENE consortium, enabling researchers to test new computational methods for polygenic risk scoring across diverse ancestry groups, while protecting sensitive health information. The software and a synthetic dataset of 6.8 million common variants and nine phenotypes for over 1 million individuals has been made publicly available.
Learn More
Published in
BIOINFORMATICS
Keywords
computational biology, statistical genetics, simulation-based inference, generative modeling, polygenic risk scoring
Synthetic data FOR GENETICS RESEARCH
HAPNEST is a new software tool that efficiently generates large synthetic datasets that closely mimic real genetics and phenotypic data. This work was carried out with the European-wide INTERVENE consortium, enabling researchers to test new computational methods for polygenic risk scoring across diverse ancestry groups, while protecting sensitive health information. The software and a synthetic dataset of 6.8 million common variants and nine phenotypes for over 1 million individuals has been made publicly available.
Learn More
Published in
BIOINFORMATICS
Keywords
computational biology, statistical genetics, simulation-based inference, generative modeling, polygenic risk scoring
BLOG
© 2025 Sophie wharrie
research highlights
Improving GeneraliSability of Health Prediction Models
Machine learning is a powerful tool for health prediction, but often struggles with generalisability challenges when making predictions for new patients. A promising strategy is to pool learning across related supervised learning tasks. A new Bayesian meta-learning approach is introduced that also models similarity between causal mechanisms of the tasks. This is applied to a case study for stroke prediction for health record and genetics data from the UK Biobank and FinnGen datasets.
Learn More
Published in
Preprint, Under review
Keywords
Meta-learning, Bayesian Hierarchical models, causal inference, deep learning, transfer learning, oOD generalisation, robust mL
Modeling disease risk in families with graph neural networks
Electronic health records (EHRs) spanning multiple generations present a new way for examining health trends in families. In collaboration with the Institute of Molecular Medicine Finland, an AI system was developed to analyze a network of over 7 million patients’ EHR data. The findings demonstrate that a geometric deep learning approach is beneficial for modeling the shared genetic, environmental, and lifestyle factors influencing disease risk in families.
Learn More
Published in
Machine Learning for Healthcare Conference (MLHC)
Keywords
graph neural networks, geometric deep learning, deep learning for sequential data, electronic health records, genetics, familial factors of disease
Synthetic data FOR GENETICS RESEARCH
HAPNEST is a new software tool that efficiently generates large synthetic datasets that closely mimic real genetics and phenotypic data. This work was carried out with the European-wide INTERVENE consortium, enabling researchers to test new computational methods for polygenic risk scoring across diverse ancestry groups, while protecting sensitive health information. The software and a synthetic dataset of 6.8 million common variants and nine phenotypes for over 1 million individuals has been made publicly available.
Learn More
Published in
BIOINFORMATICS
Keywords
computational biology, statistical genetics, simulation-based inference, generative modeling, polygenic risk scoring
research highlights
Improving GeneraliSability of Health Prediction Models
Machine learning is a powerful tool for health prediction, but often struggles with generalisability challenges when making predictions for new patients. A promising strategy is to pool learning across related supervised learning tasks. A new Bayesian meta-learning approach is introduced that also models similarity between causal mechanisms of the tasks. This is applied to a case study for stroke prediction for health record and genetics data from the UK Biobank and FinnGen datasets.
Learn More
Published in
Preprint, Under review
Keywords
Meta-learning, Bayesian Hierarchical models, causal inference, deep learning, transfer learning, oOD generalisation, robust mL
Modeling disease risk in families with graph neural networks
Electronic health records (EHRs) spanning multiple generations present a new way for examining health trends in families. In collaboration with the Institute of Molecular Medicine Finland, an AI system was developed to analyze a network of over 7 million patients’ EHR data. The findings demonstrate that a geometric deep learning approach is beneficial for modeling the shared genetic, environmental, and lifestyle factors influencing disease risk in families.
Learn More
Published in
Machine Learning for Healthcare Conference (MLHC)
Keywords
graph neural networks, geometric deep learning, deep learning for sequential data, electronic health records, genetics, familial factors of disease
Synthetic data FOR GENETICS RESEARCH
HAPNEST is a new software tool that efficiently generates large synthetic datasets that closely mimic real genetics and phenotypic data. This work was carried out with the European-wide INTERVENE consortium, enabling researchers to test new computational methods for polygenic risk scoring across diverse ancestry groups, while protecting sensitive health information. The software and a synthetic dataset of 6.8 million common variants and nine phenotypes for over 1 million individuals has been made publicly available.
Learn More
Published in
BIOINFORMATICS
Keywords
computational biology, statistical genetics, simulation-based inference, generative modeling, polygenic risk scoring
researcH highlights
Improving GeneraliSability of Health Prediction Models
Machine learning is a powerful tool for health prediction, but often struggles with generalisability challenges when making predictions for new patients. A promising strategy is to pool learning across related supervised learning tasks. A new Bayesian meta-learning approach is introduced that also models similarity between causal mechanisms of the tasks. This is applied to a case study for stroke prediction for health record and genetics data from the UK Biobank and FinnGen datasets.
Learn More
Published in
Preprint, Under review
Technical
keywords
Meta-learning, Bayesian Hierarchical models, causal inference, deep learning, transfer learning, oOD generalisation, robust mL
Published in
Machine Learning for Healthcare Conference, PMLR 2023
Technical
keywords
graph neural networks, geometric deep learning, deep learning for time series data, electronic health records, genetics, familial factors of disease
Modeling disease risk in families with graph neural networks
Electronic health records (EHRs) spanning multiple generations present a new way for examining health trends in families. In collaboration with the Institute of Molecular Medicine Finland, an AI system was developed to analyze a network of over 7 million patients’ EHR data. The findings demonstrate that a geometric deep learning approach is beneficial for modeling the shared genetic, environmental, and lifestyle factors influencing disease risk in families.
Learn More
Published in
BIOINFORMATICS
Technical
keywords
computational biology, statistical genetics, simulation-based inference, generative modeling, polygenic risk scoring
Synthetic data FOR GENETICS RESEARCH
HAPNEST is a new software tool that efficiently generates large synthetic datasets that closely mimic real genetics and phenotypic data. This work was carried out with the European-wide INTERVENE consortium, enabling researchers to test new computational methods for polygenic risk scoring across diverse ancestry groups, while protecting sensitive health information. The software and a synthetic dataset of 6.8 million common variants and nine phenotypes for over 1 million individuals has been made publicly available.
Learn More
researcH highlights
Improving GeneraliSability of Health Prediction Models
Machine learning is a powerful tool for health prediction, but often struggles with generalisability challenges when making predictions for new patients. A promising strategy is to pool learning across related supervised learning tasks. A new Bayesian meta-learning approach is introduced that also models similarity between causal mechanisms of the tasks. This is applied to a case study for stroke prediction for health record and genetics data from the UK Biobank and FinnGen datasets.
Learn More
Published in
Preprint, Under review
Technical
keywords
Meta-learning, Bayesian Hierarchical models, causal inference, deep learning, transfer learning, oOD generalisation, robust mL
Published in
Machine Learning for Healthcare Conference, PMLR 2023
Technical
keywords
graph neural networks, geometric deep learning, deep learning for time series data, electronic health records, genetics, familial factors of disease
Modeling disease risk in families with graph neural networks
Electronic health records (EHRs) spanning multiple generations present a new way for examining health trends in families. In collaboration with the Institute of Molecular Medicine Finland, an AI system was developed to analyze a network of over 7 million patients’ EHR data. The findings demonstrate that a geometric deep learning approach is beneficial for modeling the shared genetic, environmental, and lifestyle factors influencing disease risk in families.
Learn More
Published in
BIOINFORMATICS
Technical
keywords
computational biology, statistical genetics, simulation-based inference, generative modeling, polygenic risk scoring
Synthetic data FOR GENETICS RESEARCH
HAPNEST is a new software tool that efficiently generates large synthetic datasets that closely mimic real genetics and phenotypic data. This work was carried out with the European-wide INTERVENE consortium, enabling researchers to test new computational methods for polygenic risk scoring across diverse ancestry groups, while protecting sensitive health information. The software and a synthetic dataset of 6.8 million common variants and nine phenotypes for over 1 million individuals has been made publicly available.